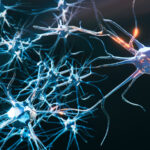
Artificial intelligence (AI) has the potential to transform healthcare as we know it. From accelerating the development of lifesaving medications, to helping doctors make more accurate diagnoses, the possibilities are vast.
But like every technology, AI has limitations—perhaps the most critical of which is its potential to potentiate biases. AI is dependent on training data to create algorithms, and if biases exist within that data, they can potentially be amplified.
In the best case scenario, this can cause inaccuracies that inconvenience healthcare workers where AI should be helping them. Worst case scenario, it can lead to poor patient outcomes if, say, a patient doesn’t receive the proper course of treatment.
One of the best ways to reduce AI biases is to make more data available—from a wider range of sources—to train AI algorithms. It’s easier said than done: Health data is highly sensitive and data privacy is of the utmost importance. Thankfully, health tech is providing solutions that democratize access to health data, and everyone will benefit.
Let’s take a deeper look at AI biases in healthcare and how health tech is minimizing them.
Where biases lurk
Sometimes data is not representative of the patient a doctor is trying to treat. Imagine an algorithm that runs on data from a population of individuals in rural South Dakota. Now think about applying that same algorithm to people living in an urban metropolis like New York City. The algorithm will likely not be applicable to this new population.
When treating issues like hypertension or high blood pressure, there are subtle differences in treatment based on factors like race, or other variables. So, if an algorithm is making recommendations about what medication a doctor should prescribe, but the training data came from a very homogeneous population, it might result in an inappropriate suggestion for treatment.
Additionally, sometimes the way patients are treated can include some element of bias that makes its way into data. This might not even be purposeful—it could be chalked up to a healthcare provider not being aware of subtleties or differences in physiology that then gets potentiated in AI.
AI is tricky because, unlike traditional statistical approaches to care, explainability isn’t readily available. When you train multiple AI algorithms, there’s a wide variety of explainability depending on what kind of algorithm you’re developing—from regression models to neural networks. Clinicians can’t easily or reliably determine whether or not a patient fits within a given model, and biases only exacerbate this problem.
The role of health tech
By making large amounts of diverse data widely available, healthcare institutions can feel confident about the evaluation, creation, and validation of algorithms as they’re transitioned from ideation to use. Increased data availability won’t just help cut down on biases: It’ll also be a key driver of healthcare innovation that will improve countless lives.
Currently, this data isn’t easy to come by due to concerns surrounding patient privacy. In an attempt to circumvent this issue and alleviate some biases, organizations have turned to synthetic data sets or digital twins to allow for replication. The problem with these approaches is that they’re just statistical approximations of people, not real, living, breathing individuals. As with any statistical approximation, there’s always some amount of error and the risk of that error being potentiated.
When it comes to health data, there’s really no substitute for the real thing. Tech that de-identifies data provides the best of both worlds by keeping patient data private while also making more of it available to train algorithms. This ensures that algorithms are built properly on diverse enough datasets to operate on the populations they are intended for.
De-identification tools will become indispensable as algorithms become more advanced and demand more data in the coming years. Health tech is leveling the playing field so that every health services provider—not just well-funded entities—can participate in the digital health marketplace while also keeping AI biases to a minimum: A true win-win.
Photo: Filograph, Getty Images